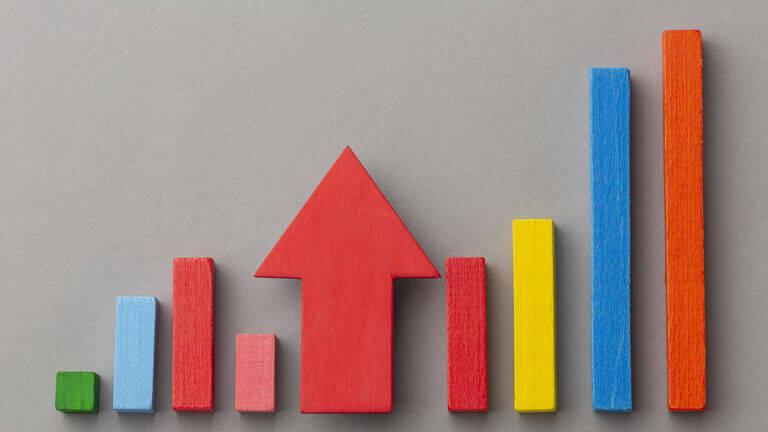
The Art of Correctly Assigning ROI
How to measure online marketing effectiveness
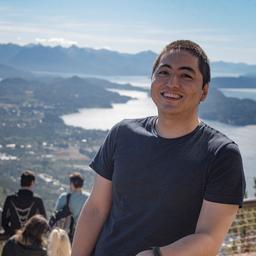
Posted by Robinson Fang
on October 15, 2024 · 7 mins read
Retail Media Networks - Part IV
👉 Intoduction
👉 Why is Attribution Important?
👉 How should you attribute?
👉 How do RMNs help attributing?
👉 Wrapping up
👉 References
Introduction
In our previous three posts, we described an overview of Retail Media Networks (RMNs) pros and cons, diving into two specific ad formats (sponsored content and display advertising) and their advertising, its functions, and its advantages.
While at the beginning of the series, we emphasized how every part of an RMN can be used to drive an uplift in sales. In this post, we will discuss effective ways to measure this uplift and, in general, online marketing effectiveness. This brings up the question:
How can we assess advertisement campaigns' impact on actual sales?
This is where attribution comes into play. Attribution is the process of linking sales to a single (or even multiple) RMN ad impressions (or, more generally, touchpoints). It is a key component when measuring campaigns' actual incremental sales lift - instead of noise.
Why is Attribution Important?
When measuring return on ad spend, you have to be able to tell which ads brought in revenue. After all, advertising is a business just like any other, and every time you invest money, you will want to understand how much ROI it generates. So even in data-lacking scenarios, you need to attribute sales to their correct source to measure a campaigns performance and optimize budgets among them. Furthermore, since specific line items and/or sponsored products can be individually measured, optimization within a single campaign can rely on accurate attribution.
A good attribution methodology does not only help to measure return on investment - it can also be a powerful analytical tool or input. For example, some products may respond better to a given placement (e.g. home banner displays) than others. If you misattribute conversions to ads shown down in the customer journey (e.g. sponsored content when listing a search) then you will end up suboptimally underspending in the real driver. In this particular example, the missed opportunity is big, as a homepage banner will probably have higher and more diverse traffic than specific search results.
Properly attributed data does make a difference when comparing messages or creatives for different types of channels or users. Any A/B testing for different phrasing strategies will suffer from mis-assignments in sales drivers, as the results may reflect effects that are not real in the tests groups, but rather the noise misattribution generates. At this point, it is clear that confidently stating "these sales can be tracked to these ads" is essential both for financially analyzing campaigns and gaining insights from them.
How should you attribute?
Now that we’ve established why attribution is essential for efficient ad strategy management, from advertisers’ point of view the next question is: how should we attribute?
When trying to grasp customers' conversion journeys, the truth is there's no silver bullet. Different stages of business growth may require different attribution models. Early-stage companies may get away with focusing on only one or two marketing channels and trying to excel at those. Yet, medium and larger companies need a more complex attribution system since they can't just rely on digital tracking of a few channels. They deal with many of them and of a different nature: sponsored influencers' posts on social media are way harder to track than typical display ads. Picking the right attribution model is a complex topic. Let us start by describing the two most simple methodologies businesses use to attribute: last-click attribution, and first-click attribution.
Last-click attribution
Say you are running a sponsored content campaign, where you list a set of items that pay to appear in relevant search results’ positions. Now, imagine that a customer makes several searches. The promoted product appears. The client clicks once or more times on the ad. They finally buy the product. This model will state that the former sale should be entirely attributed to the last click as long as the sale was made shortly after the click occurred (say, a seven-day window). In this approach, the sale will be “attributed” solely to the last click disregarding any other contribution to the user’s journey.
First-click attribution
Last-click attribution is one of many governed by a hard rule. Another example, albeit less common, is first-click attribution. Its biggest strength is its easy interpretation and understanding. The simplicity as mentioned earlier is relative to other models, since, even in these cases, tracking needs are huge. Even in these simple schemas, usually, an online, per-event tracking mechanism and the means to link specific users to this data should be set. That raises complexity on many fronts, for example, meeting privacy requirements. However, the main disadvantage comes to light as soon as we realize that clicks are not the only relevant interaction a user may have with product ads.
Multi-touch attribution
Let's stay in our sponsored content environment. What if the ad was shown to the same user several times, but was never clicked? Then -based on navigation- the site recommended the product. Finally, the user arrives at a landing page and buys it this time. Should we consider that advertising had no effect, just because of the lack of clicks? We could have a second possible interaction: ‌ ad impressions. This allows for more complicated attribution. Even in the simplest path (a print, a click, a sale) attribution can be split across interactions (usually known as touchpoints). Even more complex touchpoints could be added as not everything happens on a single channel, format, or even on the same device; there could be a path with a brand home banner, then a sponsored listing, a click, and finally a sale.
For these cases where several inter and intra-channel effects occur for any given sale, methodologies for multi-touch attribution were developed. The earlier ones used somewhat rigid rules: the linear approach assigned an equal proportion of the sale to every touchpoint the user interacted with; the U-shaped approach gave higher importance to the first and last interaction and divided the rest on the remaining touchpoints. Slightly more sophisticated, time decay approaches assign more importance to touchpoints that happened closer to the sale event than those that happened earlier on. Finally, there is a cutting-edge development with data-driven attribution. Data-driven complex models (such as neural networks or hierarchical classification approaches) get touchpoints and events as input and give their credit to a given sale as an output.
At this point, it should be clear that there is no single answer to the question of “how should you attribute”. Instead, many possible approaches are competing, each bearing its own biases and advantages but all requiring non-trivial engineering efforts for efficient tracking.
It should also be noted that an attribution model like last-click over-credits the “bottom of the funnel” channels (the ones a customer is likely to see right before their purchase) and under-credits those at the top of the funnel while first-click does the exact opposite. Even though multi-touch attribution attempts to solve this, it's important to note that all digital tracking methods describe correlation but do not imply any causality relationships between impressions or clicked ads and sales.
Combining Measurement Methodologies
A carefully designed mix of tracking, experiments, surveys, and modeling may be needed to measure marketing effectiveness properly. At Mutt, we recommend combining Online Attribution with Marketing Mix Modeling (MMM) and Lift/Incrementality studies to achieve a more accurate picture of the impact of each channel. MMM leverages historical data to estimate the incremental impact of each influencing factor (marketing activities, seasonality, etc). This is a solid complement to attribution and enables data-fueled forecasts and smart budget allocation on both online and offline channels.
How do RMNs help attributing?
Now that we know about the importance of a good attribution strategy we may return to the main topic of our blog post series: Retail Media Networks (RMNs).
It should come as no surprise that their main advantage over other ad channels is their rich data. RMNs are in a unique position to link many touchpoints to a given user; even better, they can do so without compromising privacy. In a world that is drifting towards a cookie-less stance, RMNs own first-party data gives them an outstanding advantage.
An RMN for example can link a user’s activities across many devices. Say a person searches for an item using a phone while commuting, but buys it at home using a desktop computer, being logged in both interactions allows the RMN to spot the connection between these touchpoints. Even in-store purchases could be related to ads shown in an RMN and no privacy issues would arise as everything is analyzed within the RMN’s datasets - any external report would have the PII properly removed.
Also, having real-time knowledge of the user’s purchase journey gives the RMNs an excellent position to tailor the messages shown, improving engagement and conversion rates. Maybe an item has already been on a user’s cart since yesterday? Then a banner could show a reminder to check it out and buy it. This would be impossible for any traditional ad platform to know without receiving 3rd party information.
It is worth mentioning that even though advertisers can rely on Retail Media Networks to decide on which is the right attribution model for them, RMN organizations are encouraged to provide advertisers with relevant data to integrate and execute retail media organization investment/metrics data into their media and market mix modeling (MMM) as needed. They are recommended to be transparent if they are modeling to account for sales that take place at other retailers. RMN should also avoid over-crediting and introducing noise to advertisers so as not to lose business to other competitors that are more transparent and incremental. The Interactive Advertising Bureau (IAB) and Media Rating Council (MRC) went a long way to develop precise and comprehensive Retail Media Measurement Guidelines that not only cover the topics of attribution and incrementality but also delve into the importance of transparency and how to achieve it.
Wrapping up
Attribution is a central topic when developing a successful advertising strategy. RMNs leveraging their first-party data are in a unique position to address this problem bypassing potential biases inherent in ad-platform’s tools while also respecting users’ privacy. At Mutt Data, we have plenty of experience dealing with complex tracking needs, and we have developed sophisticated attribution techniques. Check out the work we did with multi-touch attribution for Mercado Libre. Keep up with Mutt and learn about the latest developments and use cases for Artificial Intelligence and Machine Learning.
References
Follow us on LinkedIn keep up with what is coming in the future!