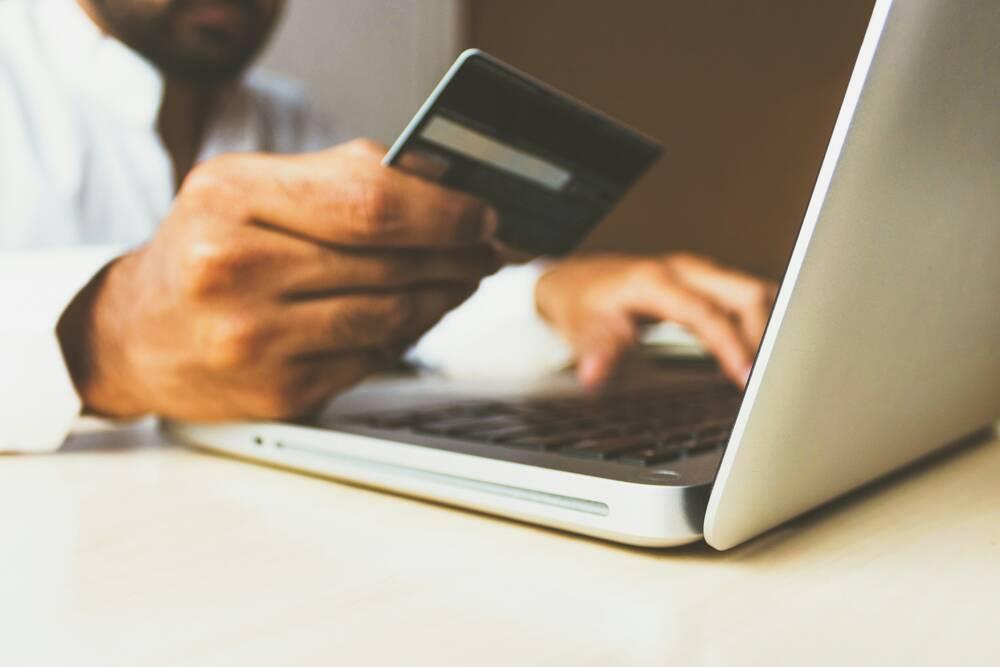
The AdTech Advantage: Boosting Mercado Pago's Customer Base
Case Study: Mercado Libre
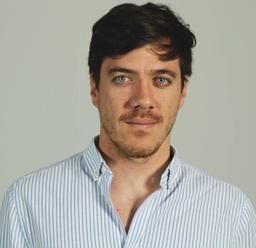
Posted by Pedro Ferrari
on March 5, 2024 · 2 mins read
About The Company
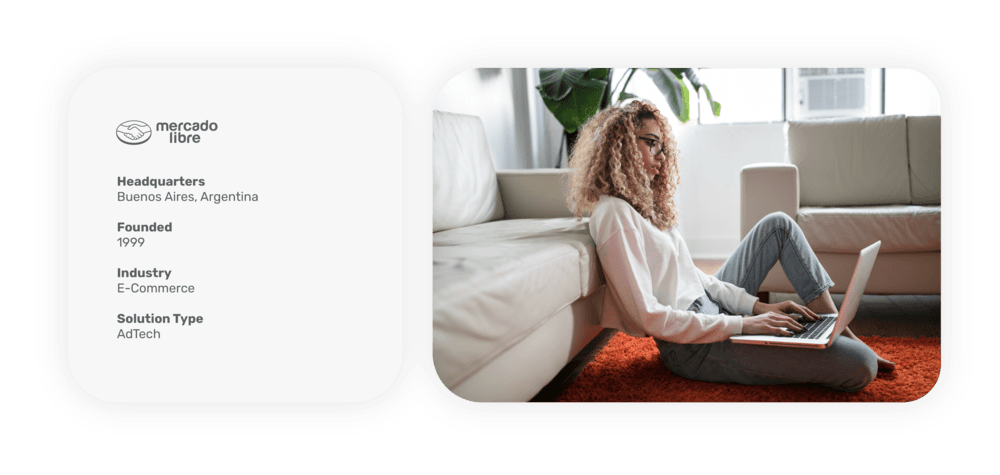
MercadoLibre is the largest online commerce and payments ecosystem in Latin America. Through a suite of technology solutions including Mercado Pago, Mercado Ads, Mercado Envios, and Mercado Creditos they enable customers in 18 countries to carry out their commerce offering solutions across the entire value chain.
Challenge
MercadoPago operates in a dynamic and ever-evolving market, competing for both buyers and sellers. To succeed in their mission to become the premier method for payments, collections and financial services among sellers, their data-driven business teams must continuously innovate and question the status quo. That's where we entered the scene to lend our expertise.
The primary objective of our project was to boost MercadoPago’s Total Payment Volume (TPV) by increasing its share of wallet. Essentially, this meant diversifying the range of products used by sellers and introducing them to services they had yet to explore.
Our close collaboration with both their business and technology teams uncovered areas ripe for improvement in their Marketing and AdTech Strategy and offering, including push notifications, emails, real estate platforms, and user segmentation enhancement.
The challenge (illustrated below) could be broken down into two main parts. Firstly, understanding what recommendations to make, and secondly, determining the most effective approach to send/implement those recommendations.
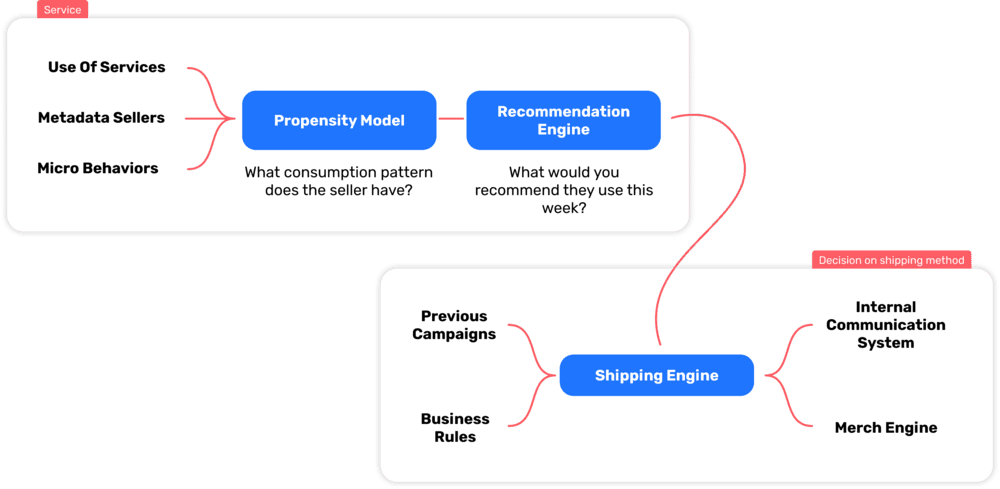
Solution
We proposed a display advertising, email, whatsapp and push-notification recommendation framework consisting of different Machine Learning models that estimates a seller's propensity to use each service in a given week, and a system that selects the recommendation matched to a seller segment.
Generated recommendations are finely tuned based on:
- When deliveries are made (balancing capping and engagement)
- Which channels are used (real estate, push, or email) depending on interaction analysis.
- Which service we should spotlight for that seller.
- Which text (copy message) to use for a specific channel or service, modelling that as a Multi-Armed Bandit.
- Analysis of previous campaigns and experiments
The recommendation system doesn’t limit itself to considering habit-based variables, it can also tailor to business objectives of different services:
- Eligibility (not all sellers can receive all messages) involves setting filters to ensure that only clients eligible for specific offers, discounts, or credits receive them based on various criteria.
- Exclusion, some sellers are excluded from all communications in order to establish a baseline for A/B testing and result comparison.
In order to build this framework, we’ve used AWS EC2 instances to build an ABT which extracts features from the existing Data Warehouse. Using specialised instances allowed us to enhance the feature-creation in the ML cycle by 3x. We’ve also used EC2 instances to host an orchestrator for our tasks, including training the ML Models and generating weekly batch predictions. With this, we could free the team's time to improve the amount of models that were being deployed on a weekly basis, from two per month to 3 per week.
Finally we use S3 as our default object storage for logging and model versioning.
Impact
Our primary business metric, which measures the number of sellers selecting MercadoPago as their primary platform, experienced a 4% nominal increase, from 6% to 10% of the seller base. Furthermore, we have incorporated monitoring best practices and tools, making it easier to track and compare metrics in real-time through user-friendly dashboards.
Testimonial
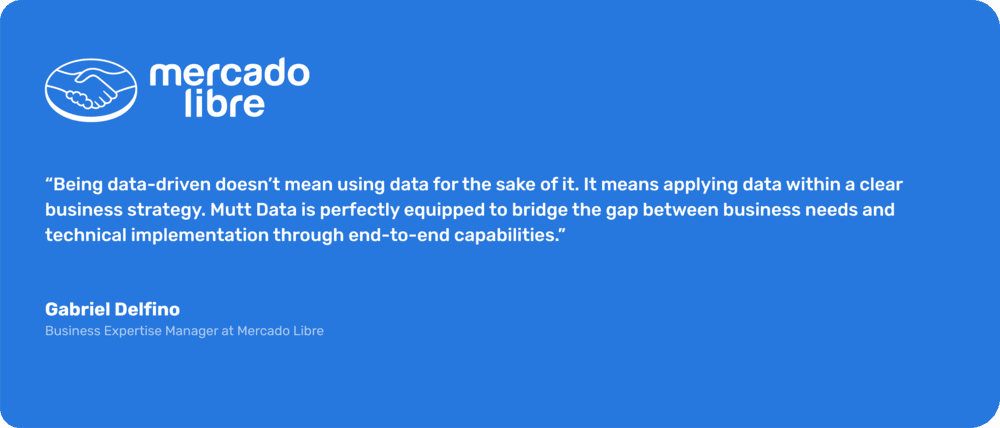