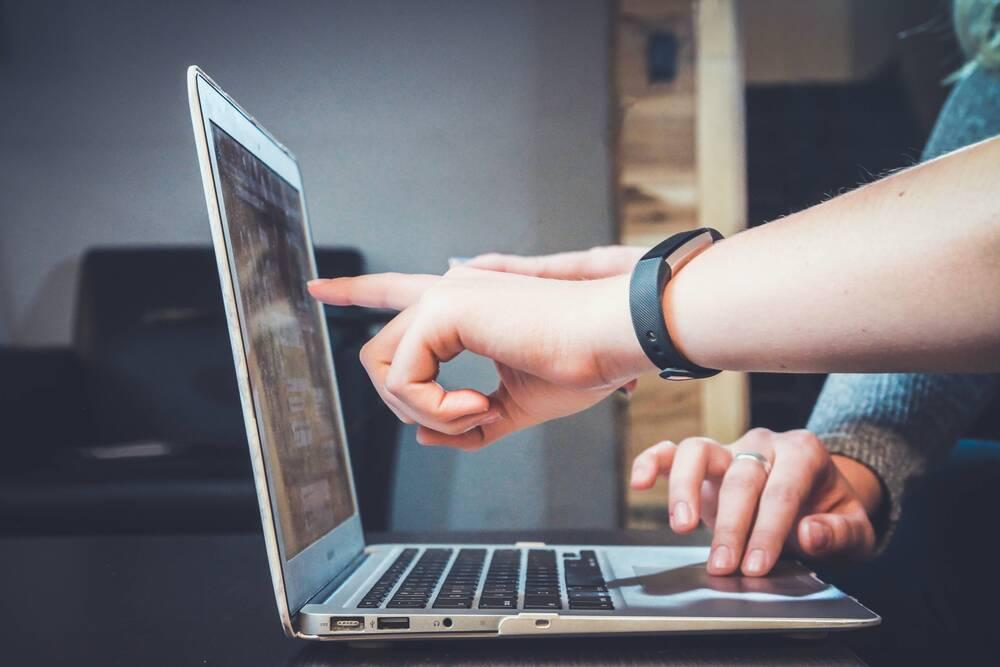
What Is Marketing Mix Modeling And How Can Automation Make It Even More Useful?
Modern Marketing
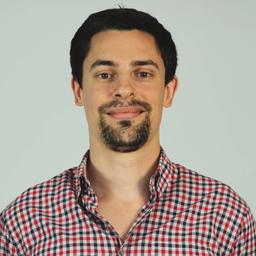
Posted by Juan Martin Pampliega
on January 13, 2022 · 8 mins read
Modern Marketing: Automated Marketing Mixed Modeling
In our second marketing post we continue to spread the word about tools and best practices we believe can take marketing to the next level. We see great potential in modern marketing best practices, we believe data science and machine learning have generated and will continue to generate opportunities to help businesses grow through transformed marketing strategies and departments. We want to be a protagonist in this transformation.
At Mutt Data we believe in efficient, automated and easily customizable systems capable of removing human-bias and the hassle of manual daily operations. Data Science is the key to modern marketing. Stay tuned for more posts about marketing best practices.
Keeping Up With Budget Allocation
Budget allocation isn't a modern concept: it's been studied for decades in traditional businesses around the world. However, although some fundamental business questions remain the same over the years the environment in which they must be answered has drastically changed. Today's markets are highly complex, and constantly changing.
As more and more ways of spending one´s marketing dollars surge and with market share, profit and customer acquisition growth being highly dependent on the use of advertising for many companies, the diversity of channels and advertising options leads to a challenge:
What is the optimal way to allocate my marketing budget in order to maximize my results? Spending too little can be as much of a problem as spending too much if our marketing dollars are not properly allocated.
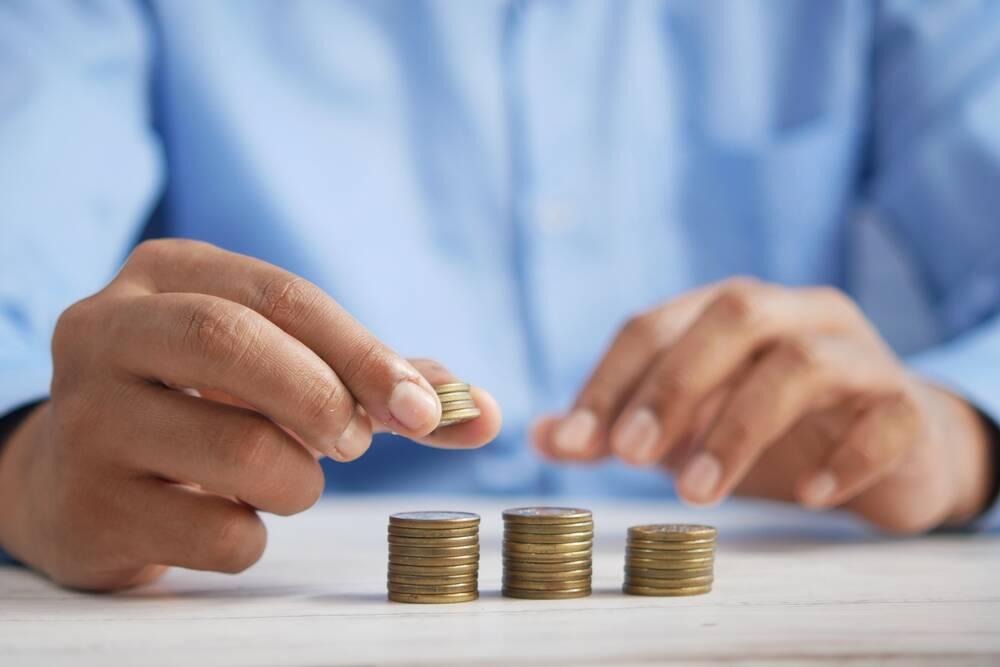
Not only has this question gotten harder to answer but companies need to answer it more frequently as well. In the past, smaller flow of information, less channels and advertising options meant budget allocation was simpler. Maybe it was revised once a year or every quarter. Today, in order to remain competitive and cost-effective, almost all companies need to adjust their allocation on a weekly, daily or even hourly basis.
So, how do we break this challenge down? Payouts, sales, return on investments are all uncertain variables. When working with a reduced number of campaigns, facing this challenge might not seem urgent. However, as the number of options grows so will the complexity of managing them.
More channels and options also means we need to understand how much each marketing input contributes to share in order to optimize how much we spend on each of these inputs. You can read more about this in our recent blogpost where we talk about multi-touch attribution.
Additionally, there are quite a few challenges to budget allocation, there is also a matter of time granularity that has to be taken into account.
Luckily, as marketing becomes more insightful and complex, so do the tools at our disposal. In recent years, the term Marketing Mix Modeling has gained a lot of attention. And, for good reason, it offers a solution to our problem at hand.
Marketing Mix Modeling (MMM) - 101
Marketing Mix Modeling is a data based statistical analysis technique which can be used to quantify the effectiveness of different components in a marketing campaign strategy in terms of a specific KPI such as sales, market share or Return on investment (ROI).
TL;DR: MMM quantifies the incremental impact of a marketing activity on predefined KPI. If I invest an additional dollar how much does it impact my KPI.
Why is this relevant? The marginal impact on our campaign, known as campaign elasticity, is not just about the difference an additional dollar has on a KPI but what that difference can tell us. Imagine a campaign where every additional dollar invested results in one dollar or less impact on sales. This result would illustrate a saturated campaign which translates into inefficient budget allocation.
This is just one example of how MMM is useful when trying to allocate budgets across different campaigns, channels or products. MMM can also be used to forecast the impact of different variations in, for example, expenditure or other variables in allocation in future campaigns.
In a not so distant past, marketers might have come by the best possible mix with excellent results. How? Intuition? Luck? The problem was replicating their strategy was a problem and so was gradually tweaking that strategy in time to cater to industry and customer trends. MMM is one more tool at marketers disposal to better understand the variables at play and their effect on ROI, sales or other specific KPIs.
So, what is behind Marketing Mix Models. Most MMMs are based on multi-linear regressions, a technique in the field of statistics used to predict the outcome of a response variable or dependent variable using various explanatory or independent variables.
For example, if we came back to marketing campaigns, the independent variables could be spending in Facebook, Google Ads or targeted discounts and the dependent variable could be sales, net profit, ROI or market share.
How Does Marketing Mixed Modeling Work?
Like most models, the common fuel to light the fire is data. Once the scope of the model and the relevant KPIs are defined data has to be collected. What kind of data? This will depend on what we are modeling but it might be related to product, pricing, sales, industry and economics. Then, Data Scientists proceed to clean and process the data. This first step is crucial, models are only as good as their data.
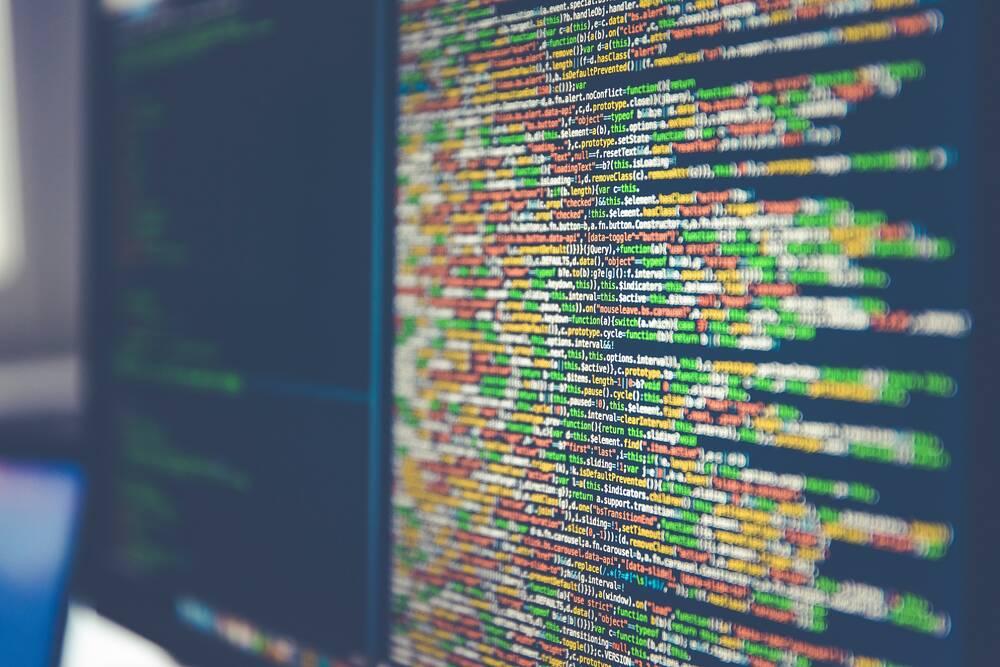
With data in the tank, data experts begin modeling. This process is dependent on scope, relevant business questions, and KPIs. MMMs ingest historical context data and analyze cost curves to predict revenue for different channels and campaigns considering constraints and spending levels.
The following figure illustrates this part of the process. The red dots represent historical data for a campaign. The area between the blue and red vertical lines is the feasible region of constraints, and finally, the fitted curve indicates revenue values and how they vary depending on changes in the spending variable.
Constraints can be set by the marketing department and may vary. Commonly they are business related requirements such as minimum or maximum spending or minimum return on investment. However, sometimes, these constraints can also be time dependent; for example, they could have different behaviours or values according to the weekday being analyzed.
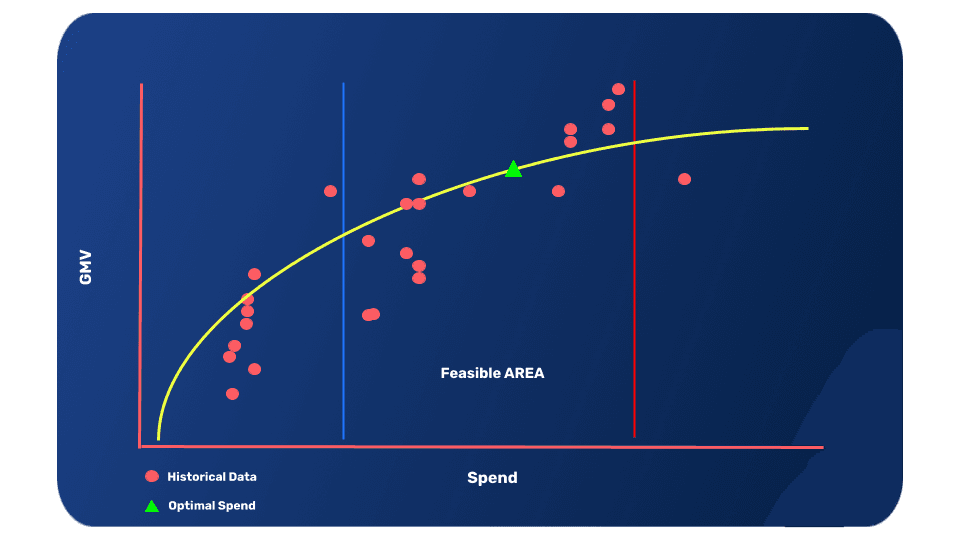
This is not a one-time task. Models are constantly tweaked, tuned, and adapted as new data inputs are introduced. Using Bayesian Linear Regression we search for the cost-revenue distribution. This model demonstrates the Bayesian framework: we form an initial estimate (a priori) and improve our estimates as we gather more data (a posteriori).
What Makes MMMs Attractive to Marketers?
Optimized Budget Allocation
A growing number of marketing channels, tools, and ways to spend our marketing dollars makes it critical to accurately measure the real profit we can expect from different channels. Modeling allows marketers to optimize their budgets and generate maximized returns on investment.
Improved Visibility / Scenario Testing
There is no perfect formula for good business but MMM allows teams to play with different scenarios, answering questions like what would happen if we pushed this channel x% or invested an additional x $ here. Better yet, if we need to achieve an x% improvement on our sales, how much more should we be spending?
Marketing Mixed Modeling vs Automated Mixed Modeling

We covered the main aspects of Marketing Mixed Modeling. What is the next step? How can automation make MMM even better? Machine Learning and automation have a key role in the next generation of Marketing Mixed Models. MMMs are not without their challenges, they can be improved upon. Here are some of the main differences between conventional MMM and Modern Automated Mixed Marketing Modeling.
One of the biggest challenges, as with many traditional models and systems is related to frequency and automation capabilities. Shifting consumer patterns and complex markets make automation today as relevant as it has ever been.
Automation plays a key role in enabling companies to react to market changes quickly. The possibility of assessing the results of changes we apply in our marketing mix quickly makes for a faster feedback loop which in turn leads to increased efficiency in our budget allocation.
Today´s newest marketing mixed models are not manual, they are automated models based on machine learning and traditional statistics. Budget allocation can be carried out at an account, multi-channel, campaign, ad-group or larger scale level. It guarantees revenue optimization through the computational solution of math problems. In addition, modern budget allocation systems can account for seasonality and trend variations automatically without the need for manual inputs or the use of dummy variables.
One way budget allocation is automated is through an exploration vs. exploitation dilemma. Budget must be allocated to different options under uncertainty. We do not know in advance which campaigns are the most profitable. So, we can define strategies that continually balance gathering information about the campaigns (exploration) and using that information to maximize revenue, (exploitation) by committing to the choice that is believed to be the best. This situation can be tested in order to leverage statistics principles to gain deeper insights.
Conventional Modeling is manual which takes longer moreover their based on static aggregate data inputs from over various years. These models don't sustain as much quality in time and make timely decision making harder as well as less frequent.
Through automation it is possible to continuously update our models with new data in a frequent and dynamic manner. By integrating with channel APIs, automated systems receive necessary performance data, centralize it, clean it and then use models to estimate curves and find the optimum allocation. The best part? Once the optimal budget allocation is found the system can automatically apply the suggested configuration without the need for analysts. Decision making becomes automated with systems, running every day or even hour of the day keeping decisions relevant and efficient.
By automating the budget allocation process human bias is reduced and by automatically tuning campaign objectives on a specified time granularity it is possible to detect under-performing campaigns in shorter periods of time. Furthermore, the frequency can be increased to daily or even hourly re-modeling or re-optimization of strategy.
Additionally, not all systems can account for lag as the effect of advertising decisions don't always impact outputs immediately. Ad Stock is a term to describe the lag effect as an advertising action builds up to its impact as well as its progressive decay.
What about the data itself? We said before that a model is only as good as it´s data. Traditional MMMs use spreadsheet data as their input, this data is gathered and integrated manually by marketing analysts. Modern systems use automatic integration. Through the use of APIs that gather granular information from a variety of different data sources it is possible to store millions of data points in databases that enable us to train better models.
Finally, validation methods are very important. Any model can be wrong. Standard MMM models use statistical validation. Currently we are seeing more and more modern models using ground truth validations to improve their accuracy. Groundtruth comes from the field of geography when satellite images were compared versus the actual terrain they were taking photos of. Similarly, in the field of machine learning, algorithms are compared versus classifications that are known in reality. A classic example is a spam filter. In the case of MMM. We can consider it to be the new gold standard in automated ml systems.
Why Mutt Data?
Our mission at Mutt Data is to help companies drive business value developing automated data products. This means we are interested in developing long term solutions that remove the daily hassle of manual, human-biased operations so that the people we help can focus on high-level decision making.
Our team of Data Nerds is highly experienced developing all kinds of automated systems for a variety of purposes. From ad platforms, real time bidders, fraud prevention solutions and attribution systems to Mix Modeling Optimization systems. We believe in adaptable and scalable solutions with user-friendly interfaces that can be used by non-technical teams.
Ideally, the person in charge of modeling should combine statistical know-how with market experience in order to understand both the technical needs of the model as well as the variables at play. At Mutt Data we work with a complementary team of data experts who come from a variety of different backgrounds: mathematicians, data scientists, data engineers, economists, marketers and statisticians.
Over the past three years our team has worked on countless projects for some of the world's largest online marketplaces, renowned financial institutions and key telecommunication companies.
Wrapping Up
We hope you’ve found this post useful, and at least mildly entertaining. If you need help figuring out the best way to boost business with data, you know where to find us.
Interested in cool content about data science and machine learning visit our blog for similar posts and more information about other internal projects like SoaM and Muttlib.